The Essentials of an Artificial Intelligence Pipe
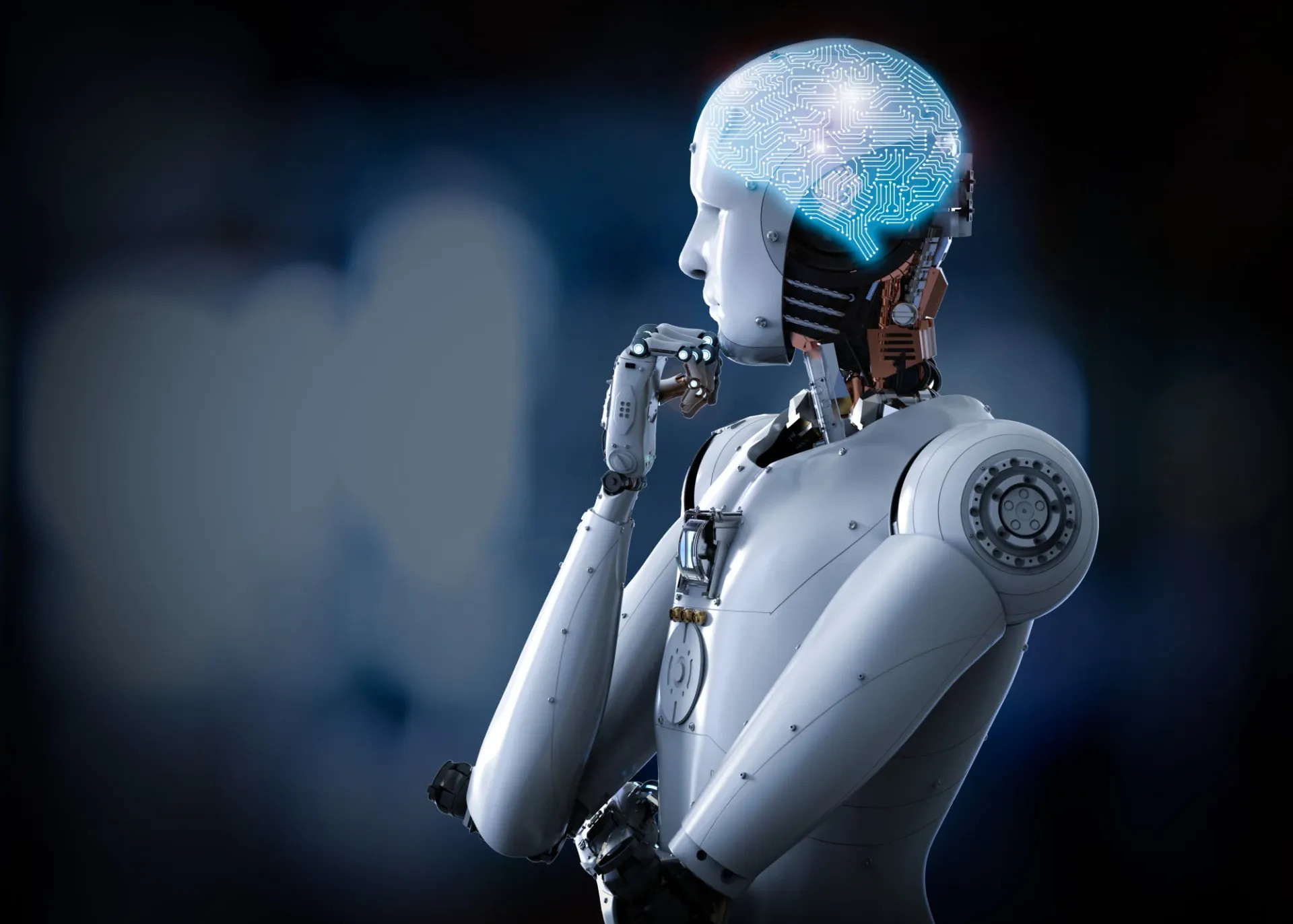
In the area of artificial intelligence, artificial intelligence has actually emerged as an effective modern technology with a large range of applications. From self-driving vehicles to personalized recommendations, machine learning pipeline are transforming various sectors. However, constructing an efficient equipment learning system requires greater than simply training a version. It includes a collection of actions, jointly called a maker discovering pipeline. In this article, we will explore the basics of a machine discovering pipeline and its relevance in the development and implementation of machine learning designs.
1. Data Collection and Preprocessing:
The primary step in a maker discovering pipeline is to accumulate and preprocess the information. The high quality and amount of the information made use of for training substantially affect the performance of the version. Data can be collected from numerous resources, such as databases, APIs, or scratching websites. It is critical to make sure that the information is relevant, depictive, and impartial.
After accumulating the data, preprocessing enters play. This action entails cleaning up the data by removing missing out on worths, outliers, and unnecessary features. Information preprocessing also consists of changing specific variables into mathematical representations, scaling the functions, and splitting the data right into training and screening sets. Preprocessing sets the structure for training a robust and accurate device learning version.
2. Version Choice and Training:
Once the information is ready, the following action is to select an appropriate machine discovering design. The selection of the design depends on the task available, such as category, regression, or clustering. There are numerous algorithms readily available, varying from easy straight models to intricate deep learning models. Each formula has its own staminas, weaknesses, and presumptions.
After choosing the version, it is time to train it utilizing the training information. Throughout the training phase, the model gains from the input data and changes its inner parameters to maximize its performance on the offered job. The training process includes feeding the input information into the model, computing the prediction error, and upgrading the design's criteria making use of optimization algorithms such as gradient descent. The goal is to minimize the forecast mistake and enhance the design's generalization ability.
3. Version Evaluation and Tuning:
Once the version is trained, it needs to be examined on cloud services to measure its performance. This is commonly done utilizing the screening established that was alloted during the information preprocessing step. Analysis metrics depend upon the particular task, such as precision, precision, recall, or mean squared error.
If the design's efficiency is not adequate, it might require further adjusting. Design tuning involves changing hyperparameters, which are settings that control the learning process of the version. Hyperparameters can influence the model's ability, regularization, or finding out rate, among other things. Tuning hyperparameters is usually done utilizing methods like grid search or arbitrary search, where various combinations of hyperparameter values are tried to find the optimal setup.
4. Implementation and Monitoring:
After selecting, training, and reviewing the version, the final step in the device learning pipe is releasing it in a manufacturing setting. Implementation involves incorporating the design into an application or system where it can make predictions on new, unseen information. This can be an internet application, a mobile app, or an automatic system.
As soon as the design is released, it is essential to continually check its performance and make required updates. This ensures that the version continues to be accurate and trusted in time. Monitoring can include monitoring prediction metrics, analyzing comments from customers, and re-training the design occasionally utilizing new information.
Final thought:
A maker learning pipeline is an organized technique to structure, training, and releasing artificial intelligence designs. It includes various phases, from data collection and preprocessing to model selection, training, examination, adjusting, and deployment. Each step plays a vital role in the success of a maker finding out task. By complying with a well-defined pipe, companies can establish robust and precise machine finding out systems that can drive purposeful insights and enable data-driven choice production. Check out this post for more details related to this article: https://en.wikipedia.org/wiki/Artificial_intelligence.